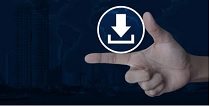
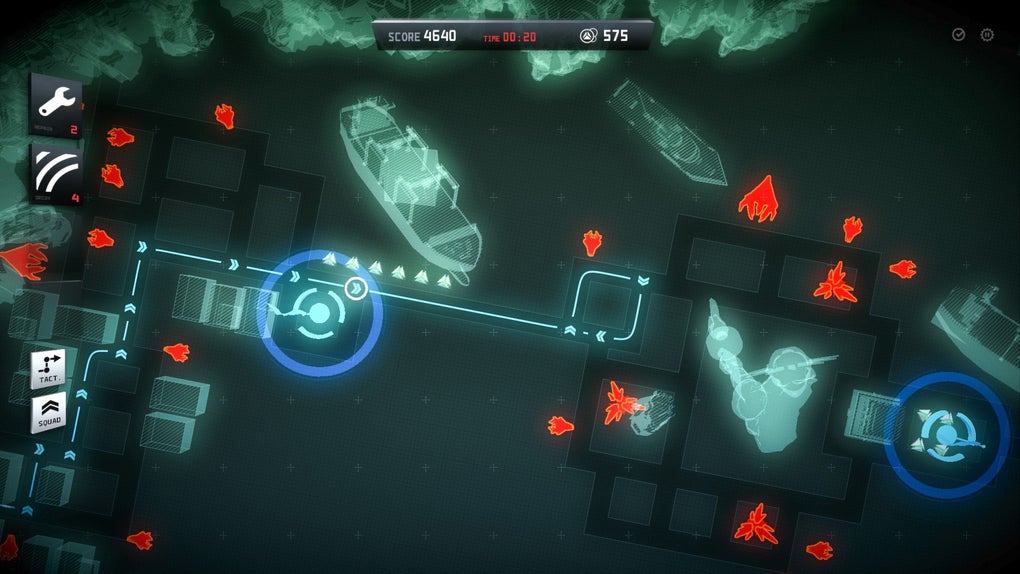
A Random Forest algorithm is developed to predict the frequency and spatial distribution of groundings while achieving an R2 of 0.55 and a mean squared error of 0.002. Massive and heterogenous datasets are well suited for machine learning algorithms and this paper develops a spatial maritime risk model based on a DGGS utilising such an approach. This paper proposes the use of the Discrete Global Grid System (DGGS) as an efficient and advantageous structure to integrate vessel traffic, metocean, bathymetric, infrastructure and other relevant maritime datasets to predict the occurrence of ship groundings.
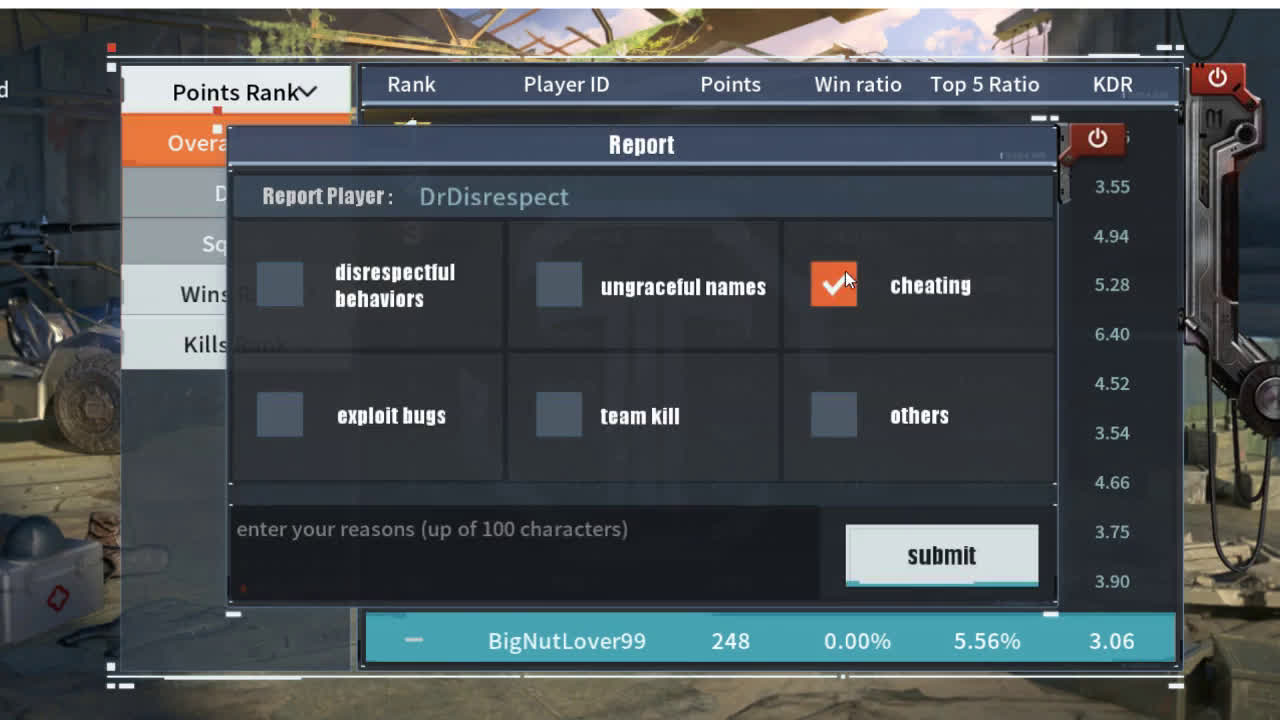
Yet, such methods necessitate the integration of large volumes of heterogenous datasets which are not well suited to traditional data structures. The promotion of navigation safety through risk reduction requires methods to assess the spatial distribution of the relative likelihood of occurrence. The abnormal behavior detection model used in the detection of ship abnormal behavior enhanced the accuracy and stability of the abnormal behavior identification and verified the validity and superiority of this method.Įach year, accidents involving ships result in significant loss of life, environmental pollution and economic losses. The simulation results demonstrated that the abnormal behavior detection model shortened the abnormal detection time. Finally, based on the public data set and the trajectory data of the inward and outward ports of ships issued by Nanjing Section, Jiangsu Maritime Bureau, the TensorFlow frame was used to establish an abnormal behavior detection model. Under the premise of introducing the attention mechanism into a GRU, the optimal GRU structure parameters were obtained through the intelligent algorithm to perform deeper feature extraction and train the ship abnormal behavior based on the optimized GRU neural network, so as to realize the detection and recognition of the trajectory data to be measured. Given the problems of complex detection methods, poor detection effectiveness, and low detection accuracy, a Gated Recurrent Unit (GRU) was proposed for ship abnormal behavior detection. It can assist maritime departments to conduct real-time supervision on a certain sea area, avoid ship risks, and improve the efficiency of sea area supervision. 46, 137.Ship abnormal behavior detection is an essential part of maritime supervision. Wippermann, F.: 1973, ‘Numerical Study on the Effects Controlling the Low-Level Jet’, Beitr. M.: 1976, ‘Validity of the Log-Linear Profile Relationship Over a Rough Terrain During Stable Conditions’, Boundary-Layer Meteorol. Longhetto, A., Anfossi, D., Fiorito, G., and Pavese, P.: 1974, ‘Asymmetries in the Horizontal Eulerian Velocity Fluctuations of the Wind’.
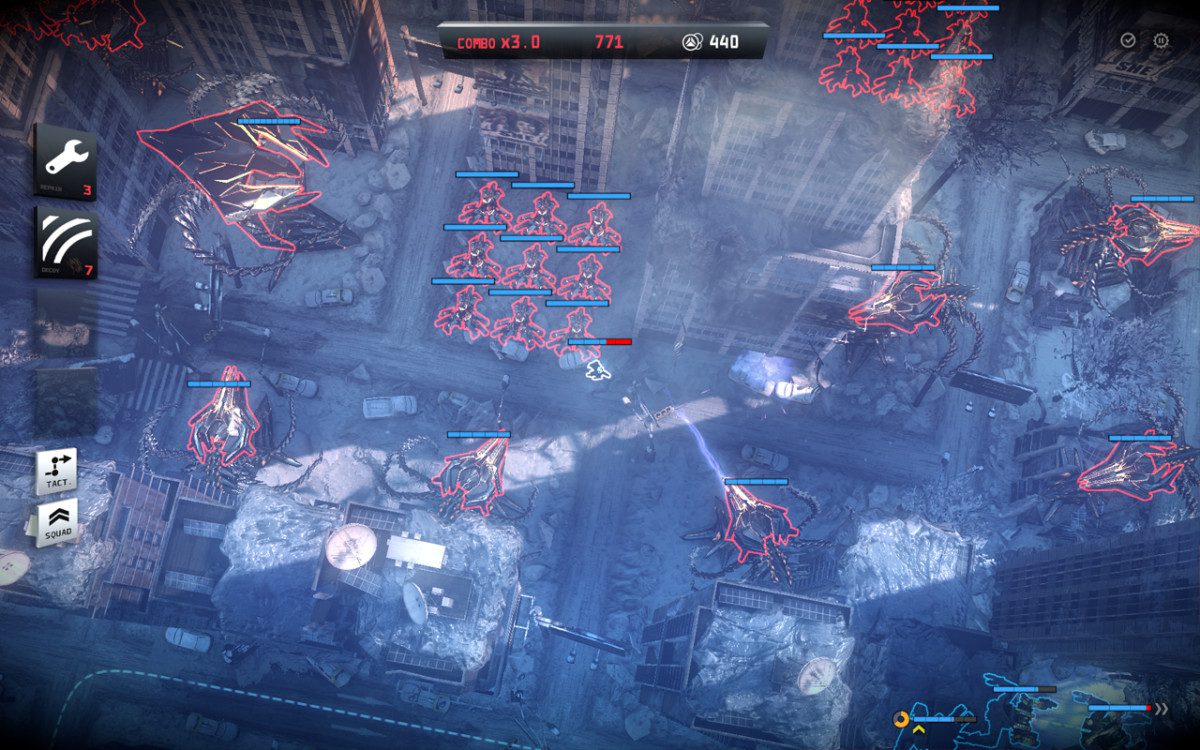
and Persano, A.: 1968, ‘A Running Average Anemograph’, Atm. and Longhetto, A.: 1973, ‘A Liquid Suspension, High-Sensitivity Anemograph’, Atm. 38, 283.īodin, S.: 1976, ‘Numerical Models of the Boundary-Layer’, ECMWF Seminars on the treatment of boundary layers in numerical weather prediction, 6–10 Sept. K.: 1957, ‘Boundary-Layer Wind Maxima and their Significance for the Growth of Nocturnal Inversions’, Bull. on Air Pollution Modeling, Frankfurt, 313.īlackadar, A. 102, 173.īacci, P., Longhetto, A., and Anfossi, D.: 1975, ‘Models of Temperature Profiles Under Convective and Inversion Conditions Connected to Air Pollution’, Proc. 8, 537.Īnfossi, D., Bacci, P., and Longhetto, A.: 1976, ‘Forecasting of Vertical Temperature Profile in Atmosphere During Nocturnal Radiation Inversions From Air Temperature Trends at Screen Height’, Quart. Anfossi, D., Bacci, P., and Longhetto, A.: 1974, ‘An Application of Lidar Techniques to the Study of Nocturnal Radiation Inversion’, Atm.
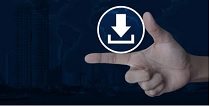